Putting smart manufacturing to work
Posted: November 8, 2024
As Wonderware’s “Suite Freaks” highlighted back in 1998, the best factories connect people with a core set of technologies.
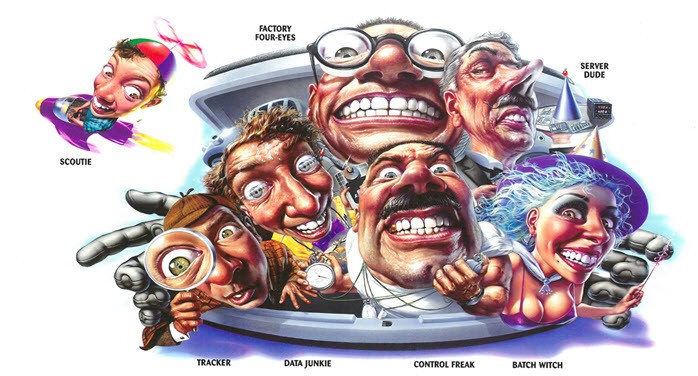
While these technologies have changed beyond recognition since then, the same core capabilities of visibility, connectivity, analytics, production management, traceability, automation, and remote access are still key to smarter factories, and smarter people working in them
Nostalgic imagery aside, the world is in a very different place than in 1998. After embracing the march to digital transformation and the autonomous plant of Industry 4.0, manufacturers have faced unprecedented disruptions, which have led to a remarkable shift in supply chain and operational thinking—back to the human-centric. As the Industry 5.0 policy brief succinctly put it, “Rather than asking what we can do with new technology, we ask what the technology can do for us.” This shift in approach is essentially what I meant by “connecting” smart manufacturing to the operating model of the factory, and it’s where smart manufacturing delivers benefits to the manufacturer.
In my previous blog Three things you can do to get value from your smart factory, I outlined the three most common missing pieces that cause smart manufacturing initiatives to stall. In this, the first of a two-part series, I’ll expand on the second point, “Connect it to your operating model,” and how that aligns with human-centric work and performance.
A major imperative behind smart manufacturing initiatives today is addressing the loss of knowledge when employees leave the company. This was recognized long before COVID with the sheer demographics of baby boomers moving into retirement. Since then, from the great resignation to quiet quitting and everything in between, the guiding question has shifted from “How can I pass the knowledge from the current to the next generation of workers?” to “How can I accelerate the contribution from workers in their first few years and encourage them to stay longer?” This shift in focus reflects the tightening labor market and rising turnover rates.
In parallel to this there have been massive investments made into the creation of digital threads through the supply chain, which provide the agility and resilience that was so obviously lacking during COVID and its aftermath. Manufacturers are now seeing that they cannot realize the full benefit of those investments without extending those digital threads through the manufacturing plants, allowing for a more realistic S&OP, and providing the visibility needed to support real-time decision-making at the supply chain level.
These drivers are at the heart of many organizations’ smart manufacturing initiatives to empower their people to improve, connect, and compare their plants, optimize operations with AI, and enable a more agile ecosystem. I’ve expanded on those four topics below and how they tie back to supply-chain agility, resilience and human-centric work.
Connect and compare
Most manufacturers work in an “infinity loop.” Production happens in the short interval while continuous improvement happens in the long interval. Although there are many flavors of this, I’ll use lean approach as a typical operating model. Under this approach, there is typically a tiered set of cadences workers in the short interval take part in, such as shift huddles, gemba walks, and daily meetings.
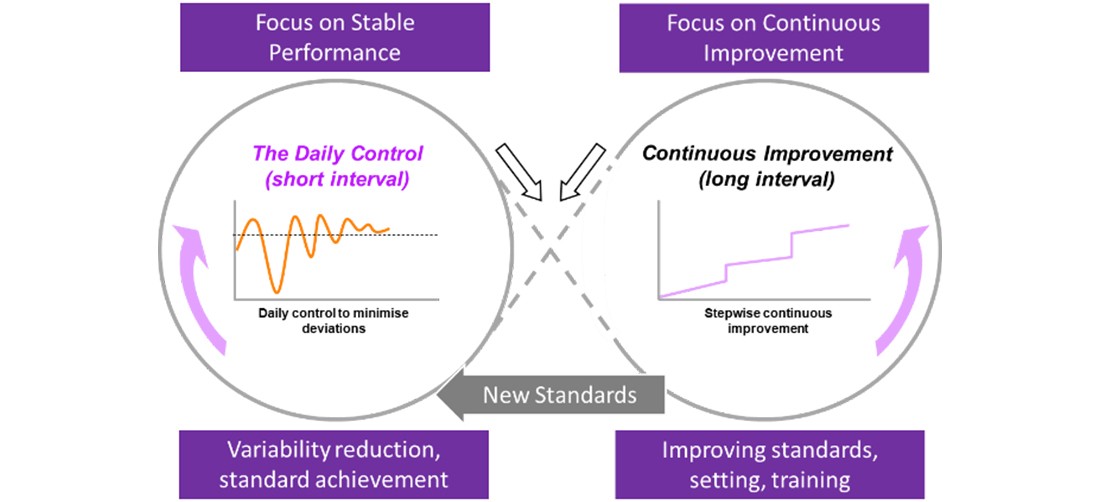
These short-interval cadences track incidents and actions up and down the hierarchy and spill over into the long-interval CI cycle. But this flow of information and actions isn’t limited to the four walls of the plant. To raise performance across the manufacturing network, work should be standardized across the plants, along with a consistent hierarchy of KPIs. By digitalizing the plant’s operating model, which provides visibility into data across locations and silos as well as accountability, manufacturers are supporting more than just their factory workers; they’re also supporting decision makers up and down the supply chain.
Empower to improve
In the same way that you can think of operations in terms of “standard achievement” (short interval) and “improve the standard” (long interval), you can apply the same thinking to improving team performance. I usually identify five key things that enable a culture of performance in manufacturing.
What are my goals? |
Production schedule, KPI targets |
How am I doing against them? |
Real-time KPI progress |
What action do I need to take? |
Andon board, scheduled actions |
Who can help me? |
Best practices, work aids, call for help, AI assistants |
How can I improve? |
Training, skills management, achievements |
The enabler for this is social collaboration that again transcends the four walls of the plant to a broader community of operators and SMEs across the business. AI can also play a part here with industrial AI assistants that are trained on your data but also have access to broader large language models (LLMs) to generate content and give recommendations. This acceleration of the mastery of skills for new workers is the second major area of value from a smart manufacturing initiative.
Optimize operations with AI
AI has quickly emerged as a leading use case for smart manufacturing, providing recommendations and optimizations that can match or exceed those of the most experienced operators. These technologies rely first and foremost on historical data to learn the relationships and context of production and process data, and then feed that learning back to the operator based on current conditions. It does this using several approaches:
Soft sensors predict a measured value from multiple other data streams that are difficult or time-consuming to measure directly. They can be used for control, quality or reporting, depending on the latency of the measurement and calculation.
Anomaly detection finds statistically significant changes in the operating envelope of a set of data streams (typically around an asset or a process). While tools like SPC provide anomaly detection for a single variable (stopping rules), anomaly detection is multivariate, combining the variability across the history of sometimes hundreds of different data points, and assessing when that whole data set is shifting statistically.
Predictive models combine some of the above elements to provide a prediction of a specific outcome, such as quality, throughput, or eco-efficiency. Either model-driven based on first principles or data-driven based on the relationships between input data streams and the modelled output (and occasionally hybrid models that use model-driven simulation data to train and correct data-driven models).
Optimization models go beyond just predicting outputs or detecting anomalies. Because data-driven models understand the relationship between input data streams and the output, they can also determine what are the most impactful streams driving the output, and what ranges they must be in to achieve a desired range of outcomes. This approach can provide operators with simple recommendations on how to run a process based on current production to improve quality, throughput, uptime, or energy use. When applied to anomaly models, they can be used to reduce variability in real time and ensure every batch is a golden batch.
Generative pre-trained transformers, or GPTs, are excellent at understanding and creating human language and other forms of responses, but they also have a drawback. To be useful, they require extensive training on LLMs. Most manufacturers are reluctant to upload all their manufacturing data into public LLMs, and even more reluctant to trust the responses to guide their operators. However, with a hybrid approach, queries that relate directly to a manufacturer’s data set are parsed internally while more general queries are prompted externally with appropriate guardrails, grounding, and security. For instance, the prompt “What issues occurred on my gizmo 3000 last shift?” might generate a dashboard of information showing downtime events and process data for the machine in question, along with maintenance information for the equipment, while a query of “What are the typical causes of motor overheating on a gizmo 3000?” might return a list of potential causes based on documents, forums, or videos that are publicly posted.
The key here is the use of AI to better sense and better respond to what is happening on the plant floor. AI can also help organizations train new plant workers more quickly. It can supplement typical onboarding training by providing insight into the current conditions in the process and guidance that equals that of your most experienced people.
These advantages, though, can easily be lost if you cannot operationalize them and make them a part of your plant’s operating model. Take for instance a typical in-house data science approach: the data scientist spends time in a plant working with operators and engineers and develops a ML algorithm that can help to significantly optimize a process. While this may work well, it typically ends up running in an Excel spreadsheet on a laptop somewhere in the plant. Once the data scientist leaves for another project, there is no one to maintain, sustain, or extend it, and moving it to another factory without also sending the data scientist is unlikely to yield any benefits. The need is not just to develop the type of algorithms outlined above, but to do so using low-code tools on a platform that is: easily integrated with your data; suited to the operators’ daily collaborations and cadences; able to replicate success from one factory to another.
Enable the agile ecosystem
One big change over the last few years is the evolution of manufacturing value chains from an almost linear supply chain view to more of a value chain network. Of course, partnering has always been common, but the role and integration of partners into manufacturing has changed dramatically. At the base of this burgeoning ecosystem is data and the capability to share data securely and rapidly bidirectionally between partners, whether that be to better utilize renewable power from your utility, working with machine builders to help them optimize their equipment, or providing current production insights to your suppliers and customers. The key enabler here is security and control over shared data, which is particularly important when large volumes of data are involved. For instance, when sharing data with a partner who provides business or supply chain analytics, it’s not ideal to duplicate data from one location to another. It’s far more advantageous to maintain the integrity of your single version of truth and provide a federated view of your data.
This also leads into the topic of multi-cloud. Most manufacturers today are operating multiple cloud platforms across their business for ERP, CRM, EAM and other uses, increasingly with a business analytics platform to join them together. While these types of platforms may be unsuitable for operationalizing smart manufacturing capabilities, it is important that the data sets leveraged for smart manufacturing are available in context to those platforms—again as a shared, federated data set, rather than a duplication of the plant floor big data.
So, these are the four objectives to connect to the daily intervals in your plant to create value from your smart manufacturing initiative:
- Connect and compare
- Empower to improve
- Optimize with AI
- Enable the agile ecosystem
Supporting these objectives requires an industrial data platform with services, connectivity, and scale. In my next blog, I’ll talk about what such a platform looks like, and the benefits of a hybrid architecture purpose-built for manufacturing that delivers effective use cases across these four objectives. I’ll also explore how smart manufacturers can take benefits gained in one plant and scale them across their manufacturing network.
Related blog posts
Stay in the know: Keep up to date on the latest happenings around the industry.