With aging assets and a time-based maintenance strategy, Sanofi sometimes experienced delays due to unexpected asset failures. It lacked essential baseline information on its equipment due to disparate data sources and manual processes. As a legacy plant, Sanofi Toronto also faced challenges in acquiring parts, and often needed engineering experts to guide repairs, again causing delays. Sanofi found it was typically taking four to seven days just to find out what had gone wrong and narrow down a root cause of a problem. By deploying AVEVA™ PI System™, Sanofi improved manufacturing capacity, reduced preventative maintenance costs, and boosted productivity.
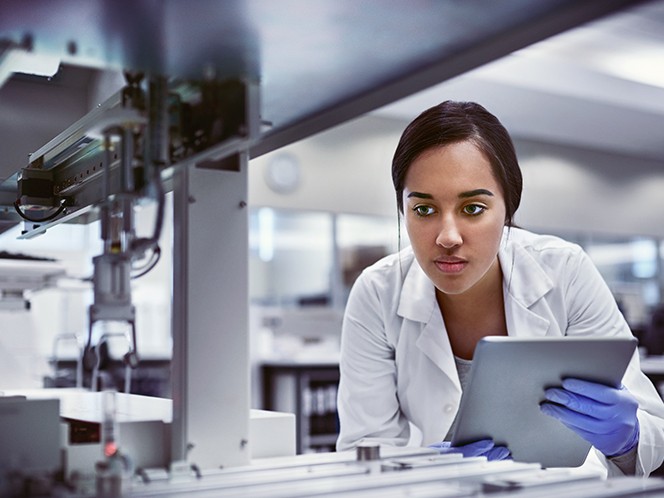
Challenges
Improving productivity by optimizing equipment performance, which was slowed by reactive and time-based maintenance
Aging assets and legacy plant created delays in fixing failures
Manual assessments and siloed data caused further delays
Results
25%
reduction in preventative maintenance costs

reduced cost of goods sold

gained productivity hours and upskilled engineering teams
“Our job is to ensure that we have a reliable supply that is maintained through our production… The function of reliability is to ensure we have a good balance, do the right kind of maintenance and ensure we have the capabilities and capacity.”
—Mathew D’souza, Site Asset Management Head, Sanofi Toronto
Understanding asset performance
Before making any changes to processes or maintenance, Sanofi needed to understand the condition of its assets and how they typically operated. The company baselined every asset in the production facility to ensure it had data for different modes, operating conditions, and phases of operation. With this information, Sanofi could begin to improve workflow planning and automation.
The company integrated AVEVA™ PI System™ and Quartic.ai as part of its reliability-centered maintenance strategy to improve equipment performance, maintenance workflow, and maintenance spend. Sanofi engineers created anomaly-detection models to discover problems earlier, ensuring workers could fix assets before they randomly failed. Sanofi also began periodic reviews of the active work orders and used machine learning to start to identify potential failures connected to different types of work orders. It also introduced physical sensors to help understand and supplement process data by adding vibration or ultrasonics sensors to critical assets and components.
Sanofi used AVEVA PI System to build a standard data and context infrastructure. With more unified data and increased automation in its workflows, planning became easier. The company made productivity gains as its staff were no longer constantly reactively responding to failures. Sanofi also gained an increased understanding of how its assets were performing. Because of the challenges related to working in a regulated environment, Sanofi couldn’t entirely eliminate time-based maintenance, but it managed to reduce the frequency and move towards a reliability-centered strategy.
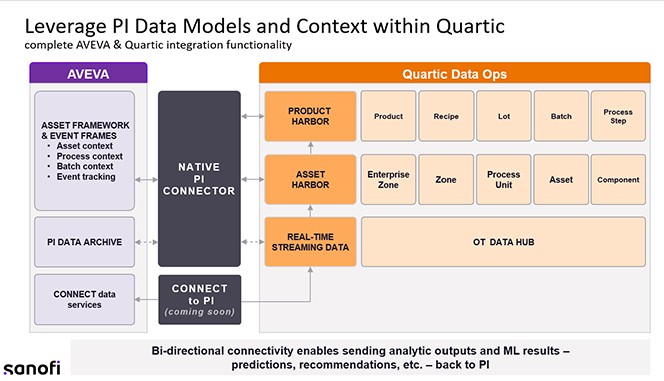
Predictive models for risk monitoring
Having successfully integrated its data and deployed anomaly detection models, Sanofi began to scale. It wanted to create more integration with its CMMS system and scale up its analysis with more machine-learning rules. With the benefits of contextualized system and sensor data in AVEVA PI System, Sanofi accelerated its use of advanced analytics.
Built on its anomaly detection engine, Sanofi developed predictive models to gain understanding of risk. Sanofi built a light risk monitoring dashboard to monitor equipment risks and establish an acceptable level of risk—was the current risk small enough to begin the next batch, or would there be a deviation? The dashboard allowed the company to be more proactive and efficient about maintenance planning and activities.
The company then tied online condition monitoring alongside deployed sensors directly into its CMMS system, automatically generating planned work orders for the asset. After full deployment of predictive tech, Sanofi is targeting reducing predictive maintenance cost by 35% by 2025. AVEVA PI System and the predictive models formed the foundation for a global e-maintenance solution, rather than the manual single site processes it had before.
“We will be leveraging what technology is out there to make our processes a little more streamlined, a little more automated, and get the most out of what is available to us.”
—Mathew D’souza, Site Asset Management Head, Sanofi Toronto
Solution
Sanofi deployed AVEVA™ PI System™ and Quartic.ai to adopt a reliability-centered maintenance approach, decreasing random manufacturing failures and reducing maintenance costs.
Scaling the vision
To build on the abilities of AVEVA PI System and Quartic, Sanofi began a risk factor trial with continuous running assets. It used a sterile air supply system, which is always running, and put in vibration and ultrasonic sensors. Engineers knew the process tags they had to look at and set target variables for average performance and room temperature—two critical parameters which, if deviated from, require a full investigation. This helped to build confidence in the risk factor shown on Sanofi’s analytics platform.
Now, Sanofi wants to scale the trial into production. The company aims to continue with its global rollout and start building user requirement specifications for new projects coming in. It aims to be best in class in terms of reliability initiatives and wants to head towards AI-managed, controlled assets. This helps ensure batches can continue if an asset is degrading, ensuring parameters can be tweaked to continue the batch or run until it has reached the end of production.
“Our target state is to get asset failure identified by a machine learning agent, that then gets verified by an engineer, and then have a series of actions that happen in parallel.”
—Mathew D’souza, Site Asset Management Head, Sanofi Toronto
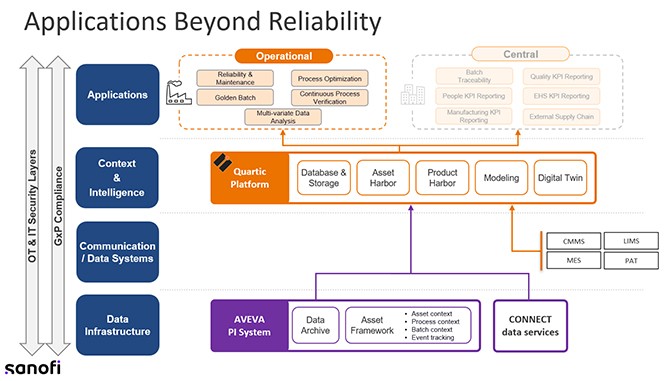
Having successfully integrated their industrial data, predictive models and IoT solutions, Sanofi is aiming for global rollout and a consistent prescriptive maintenance model. The company still has some siloed systems and is hoping to move to a single platform approach. Once its reliability program is ingrained, Sanofi hopes to apply the approach to other processes, such as batch traceability, external supply chain, and KPI reporting.
Product highlights
AVEVA™ PI System™
Collect, aggregate, and enrich real-time operations data for immediate problem-solving and easily deliver formatted data to enterprise applications and advanced analytics.